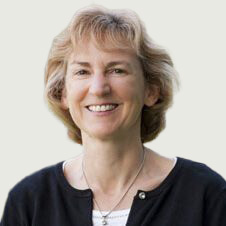
Karen Myers
Vice President, Information and Computing Sciences and Lab Director, Artificial Intelligence Center
Karen Myers, Ph.D., is the Lab Director for SRI International’s Artificial Intelligence Center.
Dr. Myers’ research focuses on intelligent systems that facilitate man-machine collaboration. Her expertise includes autonomy, multi-agent systems, automated planning and scheduling, and intelligent assistants. She has led the development of several AI technologies that have been successfully transitioned into operational use in areas that span collaboration tools, task management, and end-user automation.
Dr. Myers has served on the Executive Council for the Association for the Advancement of Artificial Intelligence (AAAI) and the International Conference on Automated Planning and Scheduling (ICAPS). She was on the editorial boards for Artificial Intelligence and the Journal for AI Research and the advisory board for ACM Transactions on Intelligent Systems and Technology. She is the conference chair for the Innovative Applications of AI conference for 2019. She was elected SRI Fellow in 2016.
Myers has a Ph.D. in computer science from Stanford University, a B.Sc. in mathematics and computer science from the University of Toronto, and a degree in piano performance from the Royal Conservatory of Music.
Publications
-
Learning Procedures by Augmenting Sequential Pattern Mining with Planning Knowledge
This paper explores the use of filtering heuristics based on action models for automated planning to augment sequence mining techniques.
-
Bridging the Gap: Converting Human Advice into Imagined Examples
We present an approach that converts human advice into synthetic or imagined training experiences, serving to scaffold the low-level representations of simple, reactive learning systems such as reinforcement learners.
-
Privacy-Aware Adaptive Scheduling for Coalition Operations
We consider the use of an advanced cryptographic technique called secure multi-party computation to enable coalition members to achieve joint objectives while still meeting privacy requirements.
-
Explanation to Avert Surprise
We present an explanation framework based on the notion of explanation drivers —i.e., the intent or purpose behind agent explanations. We focus on explanations meant to reconcile expectation violations and…
-
Semantic Instrumentation of Virtual Environments for Training
We discuss an approach in which the virtual environment is semantically instrumented in order to allow for the tracking of and reasoning about open-ended learner activity therein.
-
Demonstration-based Solution Authoring for Skill Assessment
This paper reports on an approach to creating solution models for automated skill assessment using an example-based methodology, specifically targeting domains for which solution models must support robustness to learner…