Multi-modal data analytics
SRI develops multi-modal data analytics solutions using machine learning for a variety of applications. These include image and video search, activity and fine grained recognition, social media analytics and RF signal exploitation.
Image and video search
SRI has developed the Computer Vision AI Search Tool (CVAST) for rapidly building searchable-image and user-annotation AI training databases. The CVAST tool supports ingestion and rapid object clustering and annotation of common scene object features. A flexible image/attribute database allows users to search for related features within a vast collection of image sets.
Under the DARPA Visual Media Reasoning (VMR) program, SRI created visual exploitation and indexing tools to rapidly extract mission-relevant visual intelligence from large quantities of diverse, ill-defined, unstructured imagery captured from multiple adversary sources.
Activity recognition
Under the IARPA Automated Low-Level Analysis and Description of Diverse Intelligence Video (ALADDIN) () and Deep Intermodal Video Analytics (DIVA) (TARDIS-V) programs, CVT has developed large-scale video content-retrieval systems using machine learning-based indexing of content through automatic detection of concepts comprising objects, events, actions, locations and similar.
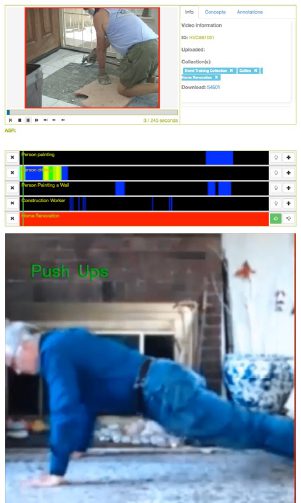
Fine-grained recognition
SRI has developed state of the art algorithms and systems for fine-grained classification of objects such as vehicles, natural objects and other classes.
Fine-grained recognition is a challenging task because small and localized differences between similar looking objects indicate the specific fine-grained label. At the same time, accurate recognition needs to discount spurious changes in appearance caused by occlusions, partial views and proximity to other clutter objects in scenes.
SRI has developed a novel multi-task deep network architecture that jointly optimizes both localization of parts and fine-grained recognition by learning from training data. We have developed memory and computational efficient algorithms for fine-grained recognition that can be easily embedded in mobile applications.
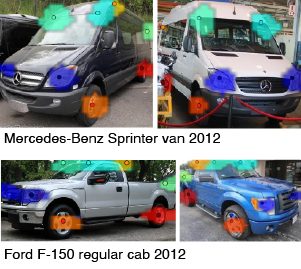
Social media analytics
Under the DARPA Social Media in Strategic Communication (SMISC), Computational Simulation of Online Social Behavior (SocSim) (SBIR M3I system), ONR CEROSS and AFRL Multimedia-Enhanced Social Media Analytics (MESA)programs, CVT has developed social media content analytics for seamless multi-way cross-platform retrieval between images, videos, text, and users using multimodal embedding of users and content in the same geometric space. Furthermore, CVT has developed a system that can detect the intent behind social media postings. Our work provides a framework for tracking the propagation of influence in social media.
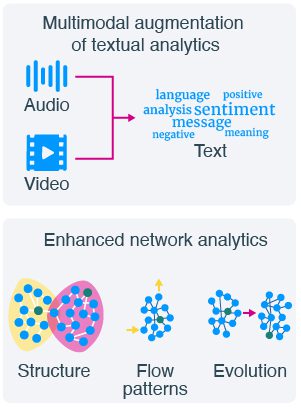
Recent work
-
Hierarchical Urban and Natural Terrain Exploitation and Reasoning
SRI is developing a system for the semi-automated geolocalization of metadata-free images and videos to find a location of interest.
-
SRI’s MatchStax Cross Platform Social media content retrieval platform
-
AURORA: Content-Guided Search of Diverse Videos
SRI is developing a novel search technology to quickly find events of interest in very large video collections.
Recent publications
more +-
Time-Space Processing for Small Ship Detection in SAR
This paper presents a new 3D time-space detector for small ships in single look complex (SLC) synthetic aperture radar (SAR) imagery, optimized for small targets around 5-15 m long that are unfocused due to target motion induced by ocean surface waves.
-
Deep Adaptive Semantic Logic (DASL): Compiling Declarative Knowledge into Deep Neural Networks
We introduce Deep Adaptive Semantic Logic (DASL), a novel framework for automating the generation of deep neural networks that incorporates user-provided formal knowledge to improve learning from data.
-
Stacked Spatio-Temporal Graph Convolutional Networks for Action Segmentation
We propose novel Stacked Spatio-Temporal Graph Convolutional Networks (Stacked-STGCN) for action segmentation, i.e., predicting and localizing a sequence of actions over long videos.