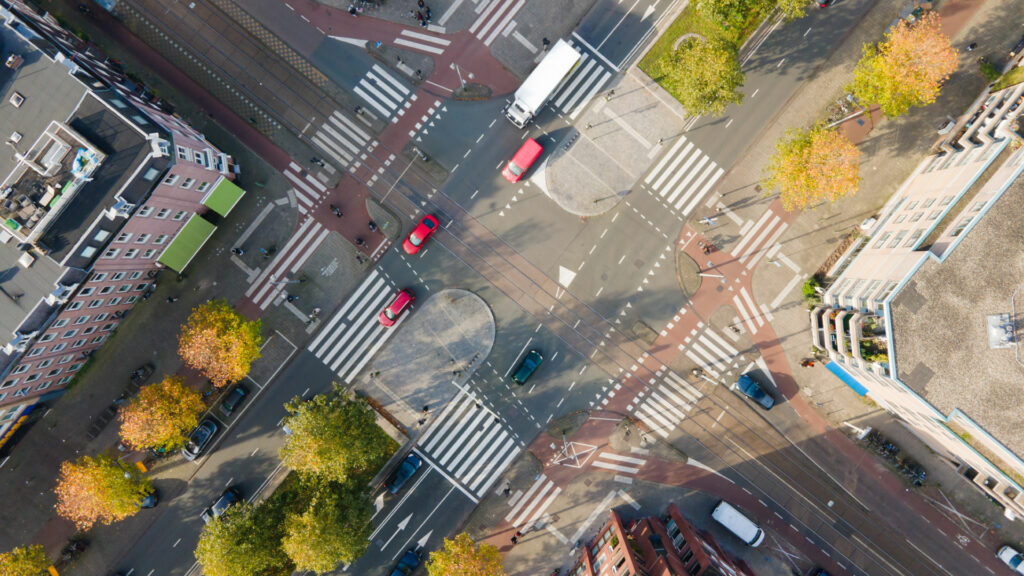
By combining ground and aerial views with computer-generated elements, users on the ground view a more accurate augmented reality experience.
Augmented reality (AR) programs, which superimpose computer-generated images over the real world, offer up applications richly ranging from gaming, such as Pokémon GO, to helping first responders navigate disaster scenes. Yet the exciting promise of the technology has been unmet so far in outdoor environments because of unreliable placement of computer-generated elements.
Now a team of experts at SRI International has developed a method for boosting the precision of element placement, overall making the experience of augmented reality smoother and more immersive in outdoor environments.
The new method developed by the SRI Center for Vision Technologies team compares ground imagery with aerial imagery from satellites. The upshot: to precisely tell where AR users are and where they are looking, technically known as geolocation and geo-pose, respectively. Getting this precise location and orientation information allows AR programs to insert computer-generated elements with higher accuracy than today’s available systems.
“By matching ground camera images to aerial satellite images, we have created a very precise visual geolocalization and geo-pose solution,” said Rakesh “Teddy” Kumar, director of the Center for Vision Technologies within SRI’s Information and Computing Sciences Division.
“The method we’ve developed greatly reduces the drift or jitter on inserted synthetic objects caused by inaccurate estimation of where AR users are located and looking,” said Han-Pang Chiu, Technical Director of the Scene Understanding and Navigation (SUN) Group at SRI, and a contributor to the AR project. “Users have a better experience because there are fewer disturbances to the illusion of the rendered and real worlds mixing together.”
Enhancing the abilities of AR programs could not only benefit humans across various commercial, industrial, military, and entertainment sectors, but also robots with visual capacities, for instance serving as autonomous vehicles or aiding search-and-rescue operations.
Where at, where to
Typically, AR programs rely on built-in device sensors and Global Positioning System (GPS) signals to locate users and gauge where their gaze is directed. However, this approach has limitations. For instance, GPS signals become patchy in the “urban street canyons” between tall buildings in cities. Big metal structures, also again buildings, can interfere with readings from sensors called magnetometers and further degrade the AR user experience.
To boost performance in urban and other settings, researchers have broadly sought to bring in “georeferenced” data, meaning sources tied to the physical environment. Pre-built databases of ground images, like those available from Google Street View, are an example. Yet these sources likewise pose limitations.
For instance, most images in ground-view databases are obtained by camera-equipped cars driving on roadways, so little data is available in off-road environments or from remote, rural areas. Collecting new images of previously unscanned areas is time-consuming and expensive. Plus, even in the most frequented, updated areas, such as urban downtowns, the ground-view imagery can be many months out of date and thus potentially inaccurate.
The view from on high
Turning to aerial imagery from satellites addresses many of these issues. The imagery covers nearly the whole of planet Earth, not just roadway-adjacent areas. Aerial imagery is also frequently updated—sometimes even daily, depending on the data source—and done so at low- or no-cost to end users.
That said, matching ground imagery to aerial imagery is no trivial task. SRI experts turned to the field of machine learning to equip their AR system to handle this matching challenge by employing a neural network—a programming arrangement consisting of connected nodes, so named because it mirrors the connectedness of neurons in the human brain.
Even more specifically, the researchers created a transformer neural network, and the first of its kind to be used in such a way for geo-localization and geo-pose determination. A transformer neural network is specially designed to weigh the significance of inputs in a manner akin to the attention humans pay (or do not pay) to information as we receive and process it.
“Our program learns very much in the way people do when it comes to the challenge of matching ground images to aerial images,” explained Kumar, who is leading the development effort of outdoor AR at SRI through an ONR project. “Humans solve the problem by getting a geometric layout of the way the world looks. So, for instance, we see a building over there and a stand of trees just past it, and we see there’s a pole on the other side of the road. Then we look for that same geometrical arrangement in the aerial image.”
To train the program, Kumar and colleagues presented it with tens of thousands of examples that both correctly and incorrectly matched ground and aerial imagery. Through this positive and negative reinforcement, the program learned to pay attention to when one patch of an image overlaps and relates to another patch of an image. Over the course of training, the AR program progressively formed an integrated, accurate representation of the ground view combined with the overhead view of the same ground.
Put to the test
The team then tried the solution in computer simulations and real-world experiments. In the former, the AR system achieved state-of-the-art performance in accurately inserting synthetic elements overtop of user views of a particular setting.
In field tests with hardware supporting the software and requirements for the AR system, results also proved promising. The researchers wore a helmet-mounted display and sensor platform tied to a backpack-mounted compact computer.
Tests were conducted in a range of environments: an urban and semi-urban area, a rural area, and a golf course. As expected, the areas without much in the way of easily recognizable humanmade structures presented more difficulty for the system to parse and place computer-generated AR imagery. Nevertheless, the results showed the feasibility of extending AR into settings literally off the beaten path.
The next steps in the development of the AR system include lowering power requirements for seamless incorporation into standard commercialized AR hardware. The system also has to be put through its paces during different times of day and seasons, as well as honed further across widely varying scenes from complex cityscapes to comparatively featureless natural landscapes.
“This is early technology, so we’re trying to robustify it, make it work in all kinds of places, and on the fly,” Kumar said. “The applications for AR are rich and varied, so it is paramount that the research and development community delivers on making the experience smooth and precise.”