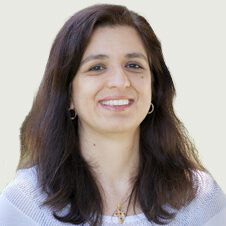
Dimitra Vergyri
Director, Speech Technology and Research Laboratory (STAR)
Dimitra Vergyri, Ph.D., research interests include information extraction from speech, voice analysis for emotional and cognitive assessment, acoustic and language modeling for languages with sparse training data, multiligual audio search, and machine translation.
She served from 2009 to 2012 as an associate editor for the IEEE Transactions on Audio Speech and Language Processing and has been part of reviewing panels and conference organizing technical committees.
Vergyri has published more than 40 papers in refereed conferences and journals. She obtained her Ph.D. degree from Johns Hopkins University in 2000.
Recent publications
-
Speech‐based markers for post traumatic stress disorder in US veterans
This study demonstrates that a speech-based algorithm can objectively differentiate PTSD cases from controls.
-
Tackling Unseen Acoustic Conditions in Query-by-Example Search Using Time and Frequency Convolution for Multilingual Deep Bottleneck Features
This paper revisits two neural network architectures developed for noise and channel robust ASR, and applies them to building a state-of-art multilingual QbE system.
-
Speech recognition in unseen and noisy channel conditions
This work investigates robust features, feature-space maximum likelihood linear regression (fMLLR) transform, and deep convolutional nets to address the problem of unseen channel and noise conditions in speech recognition.
-
Joint modeling of articulatory and acoustic spaces for continuous speech recognition tasks
This paper investigates using deep neural networks (DNN) and convolutional neural networks (CNNs) for mapping speech data into its corresponding articulatory space.
-
Toward human-assisted lexical unit discovery without text resources
This work addresses lexical unit discovery for languages without (usable) written resources.
-
Unsupervised Learning of Acoustic Units Using Autoencoders and Kohonen Nets
This work investigates learning acoustic units in an unsupervised manner from real-world speech data by using a cascade of an autoencoder and a Kohonen net.