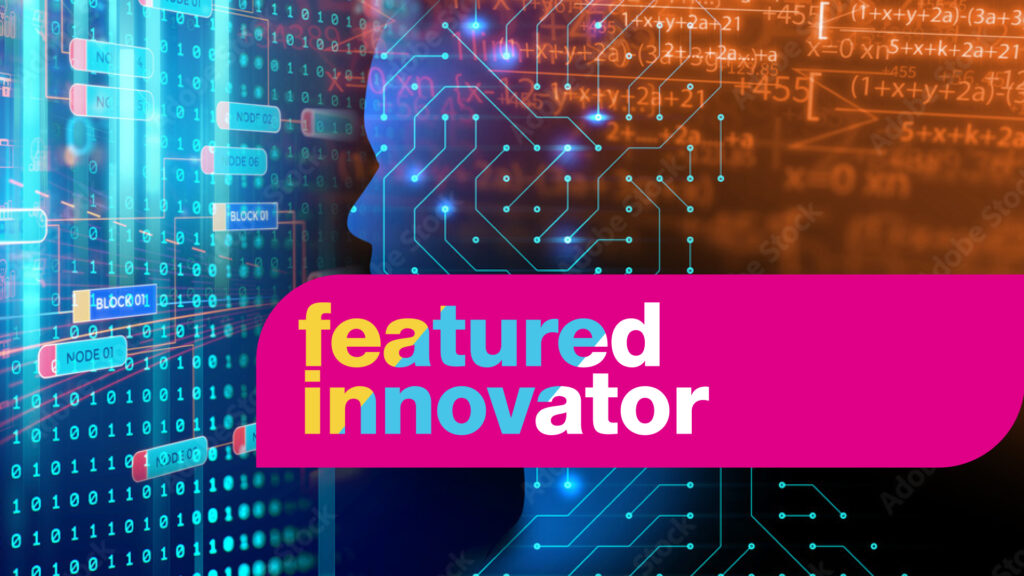
A technical director in SRI’s Computer Vision Laboratory, Yao discusses exploring new frontiers and advancing AI-enabled technology.
Dr. Yi Yao is a Technical Director in SRI Computer Vision Laboratory. Dr. Yao and her team work on a wide range of artificial intelligence (AI) and computer vision problems, including advances in explainable AI.
“When I first joined SRI, I was very impressed with the lifecycle and scope of projects. They begin with fundamental research and move outwards to deliver a deployable product: this full lifecycle approach to innovation is great to be part of,” commented Yao. She and her team focus on optimizing algorithm model training methods and ways to protect them against data poisoning attacks.
From coder to setting the scene for future developments in AI
For the first five years of Yao’s work at SRI, she was deeply involved with writing the core software to implement AI and machine learning (ML) algorithms. Her technical knowledge and well-rounded practical experience in AI resulted in a promotion to technical manager, and her success in writing grant proposals allowed Yi to set the research agenda for her group in the computer vision department at SRI International.
“For my first project, the HUNTER Project, I implemented algorithms and developed some of the user interfaces. This was an end-to-end system, and I was involved at almost every step of that system,” Yi explains.
Dr. Yao’s years of experience and involvement throughout many project lifecycles have added to her personal knowledge and deep expertise, and she is still deeply involved in ML, including deep learning (DL). Yi explains that “several years back, folks were too optimistic in terms of the capability of DL as it provides better performance than humans in tasks such as face recognition. Now, people have begun to question the validity of decisions made by DL and ML models. The ‘black box’ effect of AI means that the output of ML and DL models are not predictable.” Yi explains that developers are “sometimes overconfident in the AI output; they can give the wrong answers with 99% confidence.”
Understanding what goes on in AI’s black box is the challenge Yi Yao and her team are working on.
Decisions, decisions
The work that Yi and her team focus on helps ensure that AI is understandable, improving its accuracy, trustworthiness and predictive insight. Yi explains that a minor change in a variable can give a significantly different outcome from current AI algorithms; her team is trying to find out why. She explains that “we need a real understanding of how deep learning works and how the training works to feel confident in deploying deep models and to create robust AI-enabled technologies.”
The human in the machine
Another of Dr. Yao’s research areas is integrating human knowledge into the training of AI algorithms or the inference of a neural network. She explains: “because humans accumulate a lot of analytical models based on underlying physics, we end up with closed-form solutions. We have mathematical models to describe phenomena — the question is how to use them to train the deep neural networks.”
Dr. Yao loves to explore new frontiers, and SRI loves to innovate. This perfect match allows her and her team to explore and advance AI-enabled technologies. Dr. Yao commented, “this is a new frontier, but our team is very strong in this area. We are also able to use technologies that SRI has previously developed under government-supported programs. As always, we work to turn research into commercial opportunities.”
Projects from Dr. Yi Yao for SRI International
Some of the projects Dr. Yao has worked on/is working on include:
- Hybrid Consistency Training with Prototype Adaptation for Few-Shot Learning: an exploration of how to solve deep learning in low data regimes. Dr. Yao and her co-authors have developed new algorithms to bridge data gaps in deep learning training.
- Confidence Calibration for Domain Generalization under Covariate Shift: research into how model calibration addresses the problem of confidence calibration for domain generalization.
- Improving Users’ Mental Model with Attention-directed Counterfactual Edits: exploring the need for effective approaches to improve the end user’s mental model of the deep neural network-based AI systems.
- Trigger Hunting with a Topological Prior for Trojan Detection: addresses the problem of identifying Trojaned training models, i.e., algorithms impacted by poisoned data.
Making innovation fly!
Dr. Yao is a creative thinker who has found a home at SRI. She told us that at the time, she was surprised to see how complex the problem space of the project was. However, as time passed and her expertise grew, difficult challenges seemed less so. “As I continued to work at SRI, my surprise level at extremely difficult challenges of the work I do becomes less as the threshold gets higher and higher. It’s now difficult for me to get surprised by some of the project challenges, even though they are extremely difficult to solve. SRI has given me the wings to fly and allow my creativity to be applied to real-world problems.”