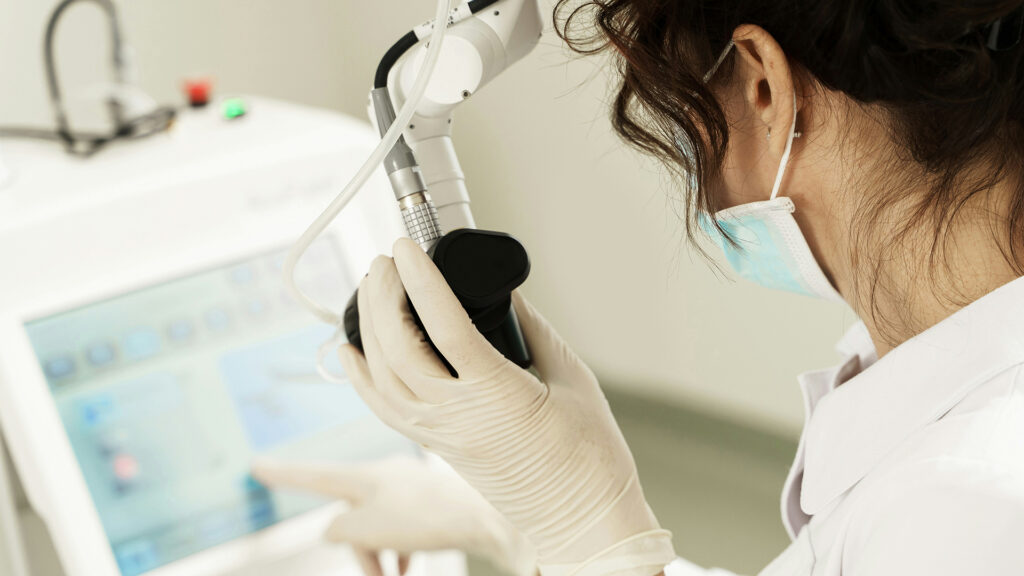
DASL combines machine learning and human expertise to provide insights for a variety of applications.
SRI researchers are taking a new approach to AI and have built a system that brings more answers and information to experts in their fields using data sets that don’t require enormous amounts of data for training. A project dedicated to this effort is Deep Adaptive Semantic Logic, or DASL (pronounced “dazzle”), a kind of next-generation AI.
This new approach would mean that chemists could explore new drugs using the knowledge that has been collected by chemists from all over the world. Doctors could potentially understand more about a rare kind of cancer that others may have more experience with on the other side of the continent. Climate scientists working in specific regions using specific technologies can benefit from one another’s work and findings.
In fact, the examples can apply to just about any industry to solve big problems. At DASL’s foundation is an AI model that combines learning with reasoning.
Key to DASL is the combination of the AI modalities of machine learning and logic-based automated reasoning with human knowledge. In practice, this hybrid approach involves building rules borne of human expertise into specific applications. Thanks to augmented machine learning, DASL does not waste time and resources computing every single possibility. It stands in direct contrast to today’s mainstream AI belief that bigger is better, where massive computations plow through the largest possible data sets to train trillions of AI-model parameters.
DASL adheres to a “work smarter, not harder” mantra
“DASL helps us build powerful AI without the need for big infrastructure, electricity burn, and time,” said John Byrnes, associate technical director in the Advanced Analytics group of the AI Center at SRI. “Ultimately, DASL can produce custom business solutions for specific applications, even where small amounts of data are available, or where much of the available data is noisy, while also needing less resources to do its job.”
In development at SRI for years, DASL has seen early applications in fields including predictive maintenance, drug discovery, disaster relief, national security, healthcare diagnostics, supply chain management, and insurance. Its bespoke approach of constructing a model guided by rules pertinent to a company’s requirements bucks the trend of general-purpose, generic big-data AIs.
DASL is in use today at two startups that spun out of SRI: DecodedHealth for healthcare diagnostics and Synfini for drug discovery and advanced material research.
“DASL provides an alternative vision of the future of AI, where people don’t need vast amounts of data to address complex challenges,” said Byrnes. “Solutions can instead be generated using custom-tailored, small-data AI that ‘speaks the language’ of specific applications.”
DASL in action
For a practical manifestation of DASL incorporating human expertise, Byrnes points to drug discovery. Say chemists at a pharmaceutical company are seeking a new cancer-fighting compound based on an intriguing, small set of initial compounds. Synthesizing more advanced versions of similar compounds one at a time would be labor-intensive and costly, so chemists turn to AI to virtually synthesize compounds of interest.
Ordinarily, a conventional machine learning model for drug discovery would have to spend weeks of expensive compute power grinding through potentially millions of trial-and-error configurations to arrive at a handful of new candidate compounds worthy of more detailed study.
“DASL helps us build powerful AI without the need for a big infrastructure, electricity burning, and time.” – John Byrnes
With DASL, a model can instead be built with fundamental rules or “language” of chemistry alongside application-relevant human knowledge — for instance, a broad category of molecular substructures may not be usable because of toxicity levels. By bringing together this expert medicinal chemistry knowledge with a small data set of initial compounds, DASL could make viable recommendations for new compounds.
“In this example, the chemists have expert knowledge, but perhaps not enough to solve the whole problem, and they’ve got important data, but not enough to solve the whole problem,” said Byrnes. “Examples like this can be addressed using DASL to leverage the data and the human knowledge in a unified framework.”
Importantly, the solution generated using DASL is not intended to be a one-off from initial model creation; rather, clients can work with DASL to input emerging parameters based on DASL’s data. For the drug compound example, clients might want the model to preserve, vary, and optimize a set of promising substructures identified prior.
How DASL does it
At its core, DASL is a series of neural networks that train themselves with the guidance of human expertise. Because of that guidance, DASL takes much of the guesswork out of machine learning. In today’s brute force approaches, the gaps between a generic, large dataset and user-requested answers are determined by arbitrary guesses consistent with the data. Bad guesses may also be consistent with small data sets, but they won’t be used due to the expert knowledge that DASL incorporates in the model.
Byrnes offers a simplified, but illustrative example of a weather model being asked to predict rainfall based on historical records and current meteorological data. It is reasonable that hourly and daily rainfall are predicted by separate models, but the models need to be consistent. If one model predicts that it’s going to rain an inch in the morning, an inch in the afternoon, and an inch at night, then the model shouldn’t predict zero inches for the day.
“DASL doesn’t eliminate wrong guesses, but it avoids many of them by filtering out inconsistent ones with what humans add to the model,” said Byrnes.
Transparent, explainable AI
Constructive iteration using DASL could offer transparency and explain its reasoning in natural language, based on rules provided by humans. When DASL gets something wrong through faulty reasoning, users can see the reasoning and correct it, thereby enhancing the model for clear insights, Byrnes explained.
Along with his colleagues at SRI, Byrnes is continuing the development of custom DASL use cases in collaboration with client subject matter experts to solve specific problems. Overall, DASL is blazing a path forward for AI that tackles specific and complex problems, all while consuming fewer resources — setting the stage for AI to be tailored and sustainable.
“To develop the next generation of AI, we are going to have to think big and small,” said Byrnes.