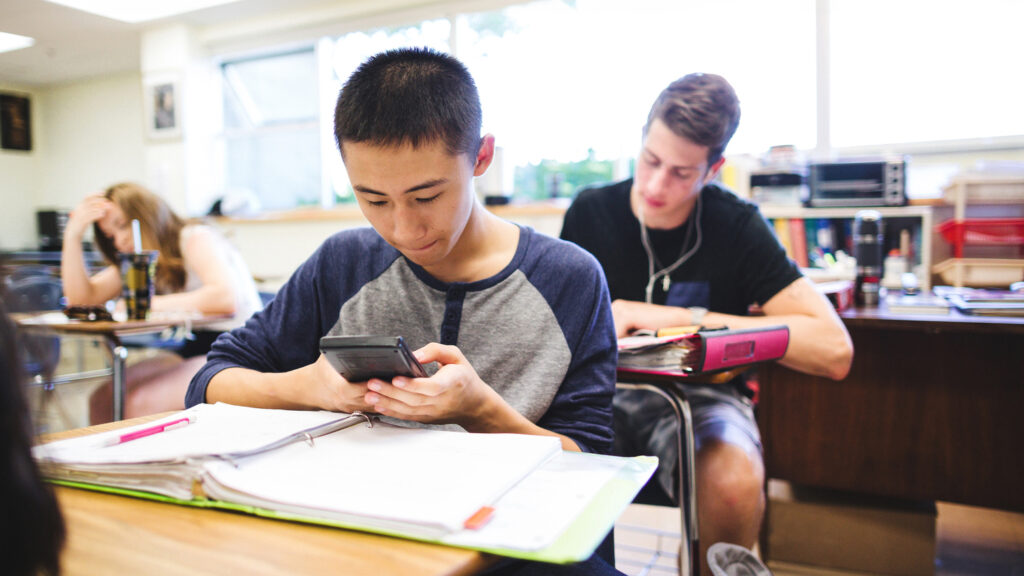
An intelligent anonymization system for individualized education programs (IEPs) could support best practices in special education.
In the United States, an IEP is a legal document prepared for every child in a public school who needs special education services. Beyond basic demographic information, IEPs describe students’ strengths, disability-related needs, and school performance, and based on these evaluations, provide a specialized and individualized instruction plan, accommodations, and services for that child.
IEPs are the main tool schools use to document students’ special education services and progress. Yet due to their narrative-based format and privacy laws that limit the sharing of information, IEPs cannot be readily compared and analyzed across students with disabilities to identify best practices and improve outcomes.
To address this, SRI is developing an automated redaction program using AI techniques to anonymize identifying information from IEPs and to analyze text-based information using natural language processing. Currently, such redaction is done in a manual, time-consuming, error-prone, limiting manner. But making large numbers of IEPs available for study through auto-redaction coupled with AI-enabled coding of IEPs into databases could power deep analyses and potentially boost the efficacy of special education.
SRI researchers are applying machine learning and natural language processing to build a redaction program initially geared toward PDF files
“My engagement with school districts around the country has shown that there is a great need for better data in special education,” said Adrienne Woods, a senior education researcher with the Center for Learning and Development at SRI. “That got us thinking about how we could leverage technology advances in AI to utilize the data that schools are already mandated to collect in individualized education programs.”
“Our goal,” Woods continued, “is to reduce barriers to accessing and using this important data. We hope this will improve outcomes for students with disabilities.”
Addressing challenges in special education
Woods is leading research dedicated to this goal in collaboration with Dayne Freitag, the Technical Director of the Advanced Analytics group in SRI’s AI center. Woods brings her expertise in special education, while Freitag and colleagues bring expertise in applying AI to data science mining and integration.
Woods’ interest in this research area traces back to childhood when her younger brother received special education services. Going through the special education system posed new challenges with each school year for Woods’ family, and overall, she and her brother had very different experiences.
For one of her projects while at the University of Michigan for her PhD, Woods went into a local school district to speak with parents, teachers, principals, and other stakeholders about their special education programs. Woods found that in general, policy decisions were made with the full intention of being helpful or remediating perceived issues in the special education system. However, these decisions oftentimes were based on insufficient data to fully understand the problem, and as a result, had the unintended consequence of hindering schools in achieving their mission.
“Parents and teachers want children to succeed,” said Woods. “It is very difficult when student information is unclear or lacking. We all need better data.”
Gaining new insights
Challenges abound. For starters, although IEPs generally contain the same information, the document formats vary significantly from school to school, let alone state to state. For proper redaction, an AI program must be able to dynamically understand these variances.
SRI researchers are applying machine learning and natural language processing to build a redaction program initially geared toward PDF files, a common IEP file type. The anonymized data regarding student disabilities and learning methods, along with strategies and instructions for teachers and special education providers will then be coded by AI programs into what Woods calls “a living data system” that grows each year as new IEPs are produced.
“We’re envisioning this as a very flexible tool to fit each school districts’ needs,” said Woods. “Teachers, administrators, policymakers, and researchers can see anonymized data sets within or across districts and states to understand who may need more professional development, where funding should be invested, which districts may be models of success, and so on.”
Helping each student thrive
One promising modality Woods and colleagues are exploring is predictive modeling of individual student responses to special education interventions (referred to as treatments and quantitatively as dosages, like in healthcare). This data science-driven approach collects IEP information to predict how well students are going to do in response to treatment given their profiles of strengths and unique needs, informed by wide comparisons to students who have received similar treatment with similar doses.
“We can start to chart trajectories that we expect students to follow,” said Woods. “This way, educators can make timely course corrections if students are not following that trajectory, rather than waiting until the end of a school year. And at the district level, administrators can be more forward-thinking in terms of staff planning and resource management.”
[The research team published an article on IEP data for practitioners or anyone who works with IEPs.]