Citation
Pedro Sequeira, Melinda Gervasio; Proceedings of the 1st World Conference on eXplainable Artificial Intelligence (xAI 2023). To appear.
Abstract
In recent years, advances in deep learning have resulted in a plethora of successes in the use of reinforcement learning (RL) to solve complex sequential decision tasks with high-dimensional inputs. However, existing systems lack the necessary mechanisms to provide humans with a holistic view of their competence, presenting an impediment to their adoption, particularly in critical applications where the decisions an agent makes can have significant consequences. Yet, existing RL-based systems are essentially competency-unaware in that they lack the necessary interpretation mechanisms to allow human operators to have an insightful, holistic view of their competency. Towards more explainable Deep RL (xDRL), we propose a new framework based on analyses of interestingness. Our tool provides various measures of RL agent competence stemming from interestingness analysis and is applicable to a wide range of RL algorithms, natively supporting the popular RLLib toolkit. We showcase the use of our framework by applying the proposed pipeline in a set of scenarios of varying complexity. We empirically assess the capability of the approach in identifying agent behavior patterns and competency-controlling conditions, and the task elements mostly responsible for an agent’s competence, based on global and local analyses of interestingness. Overall, we show that our framework can provide agent designers with insights about RL agent competence, both their capabilities and limitations, enabling more informed decisions about interventions, additional training, and other interactions in collaborative human-machine settings.
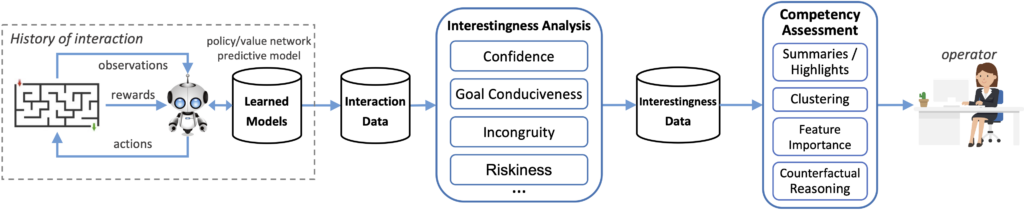